ENERXICO is developing a computer code called Black Hole (or BH code) for the numerical simulation of oil reservoirs, based on the numerical technique known as Smoothed Particle Hydrodynamics or SPH. This new code is an extension of the DualSPHysics code and is the first SPH-based code that has been developed for the numerical simulation of oil reservoirs and has important benefits versus commercial codes based on other numerical techniques.
The BH code is a large-scale massively parallel reservoir simulator capable of performing simulations with billions of “particles” or fluid elements that represents the system under study. It contains improved multi-physics modules that automatically combine the effects of interrelated physical and chemical phenomena to accurately simulate in-situ recovery processes. This leads to the development of a graphical user interface multiple-platform application for code execution and visualization, and for carrying out simulations with data provided by industrial partners and performing comparisons with available commercial packages. Furthermore, a large effort is being made to simplify the process of setting up the input for reservoir simulations from exploration data by means of a workflow fully integrated in our industrial partners’ software environment.
An oil reservoir is composed of a porous medium with a multiphase fluid made of oil, gas, rock and other solids. The aim of the code is to simulate fluid flow in a porous medium, as well as the behavior of the system at different pressures and temperatures. The tool should allow the reduction of uncertainties in the predictions that are carried out. For example, it could answer questions about the benefits of injecting a solvent, which could be CO2, nitrogen, combustion gases, methane, etc. into a reservoir, and the times of eruption of the gases in the producing wells. With these estimates, it can take the necessary measures to mitigate their presence, calculate the expense, the pressure to be injected, the injection volumes and most importantly, where and for how long. The same happens with more complex processes such as those where fluids, air or steam are injected, which interact with the rock, oil, water and gas present in the reservoir. The simulator should be capable of preparing monitoring and measurement plans.
In order to be able to perform a simulation of a reservoir oil field, an initial model needs to be created. Using geophysical forward and inverse numerical techniques the ENERXICO project will evaluate novel, high-performance simulation packages for challenging seismic exploration cases that are characterized by extreme geometric complexity. We will particularly explore high-order methods based upon fully unstructured tetrahedral meshes and also tree-structured Cartesian meshes with adaptive mesh refinement (AMR) for better spatial resolution. Using this methodology, our own packages, commercial packages, and seismic and geophysical data of naturally fractured reservoir oil fields from some of our commercial partners, we will be able to create the geometry (see Figure 1), and properties (see Figure 2) of the oil reservoir field we want to study.
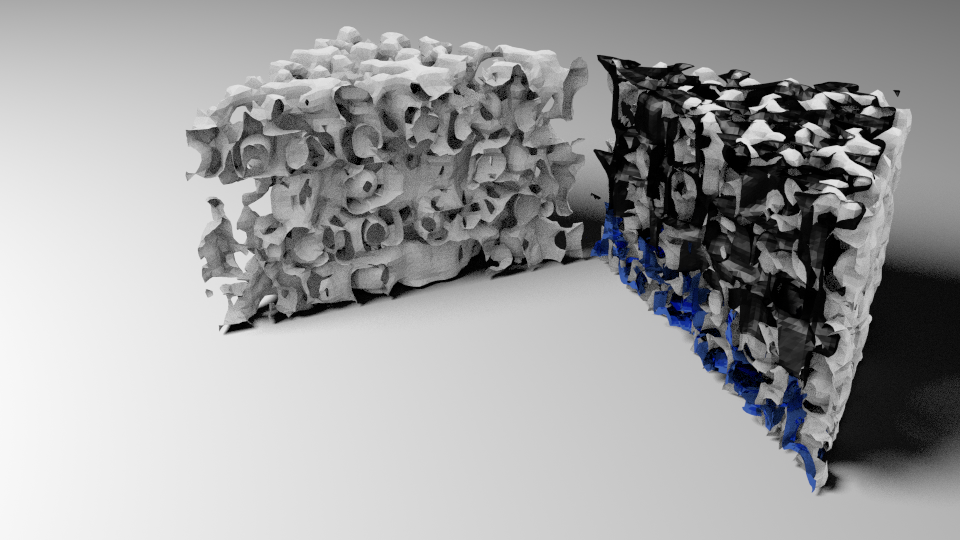
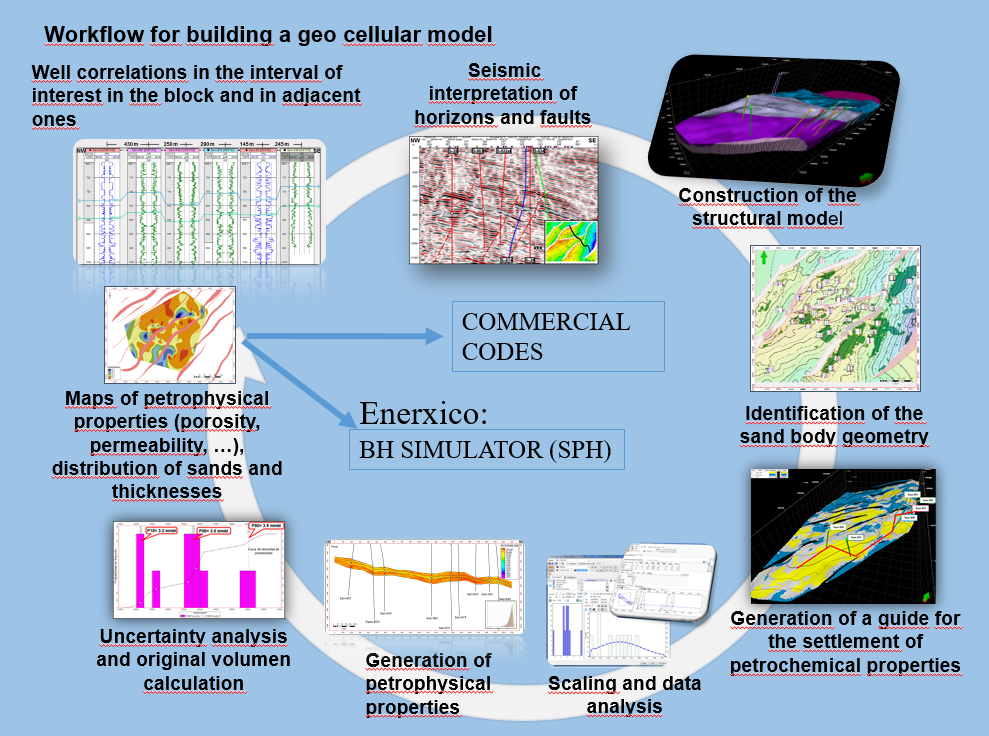
The SPH numerical simulations will then be compared to the results obtained from commercial codes, from multiphase laboratory experiments (see Figure 3) and field data from some of our commercial partners. There are many uncertainties in the system—from seismic and geophysical data to numerical simulations and the field data information. We are developing an artificial intelligence (AI) system to reduce the uncertainties in these numerical simulations.
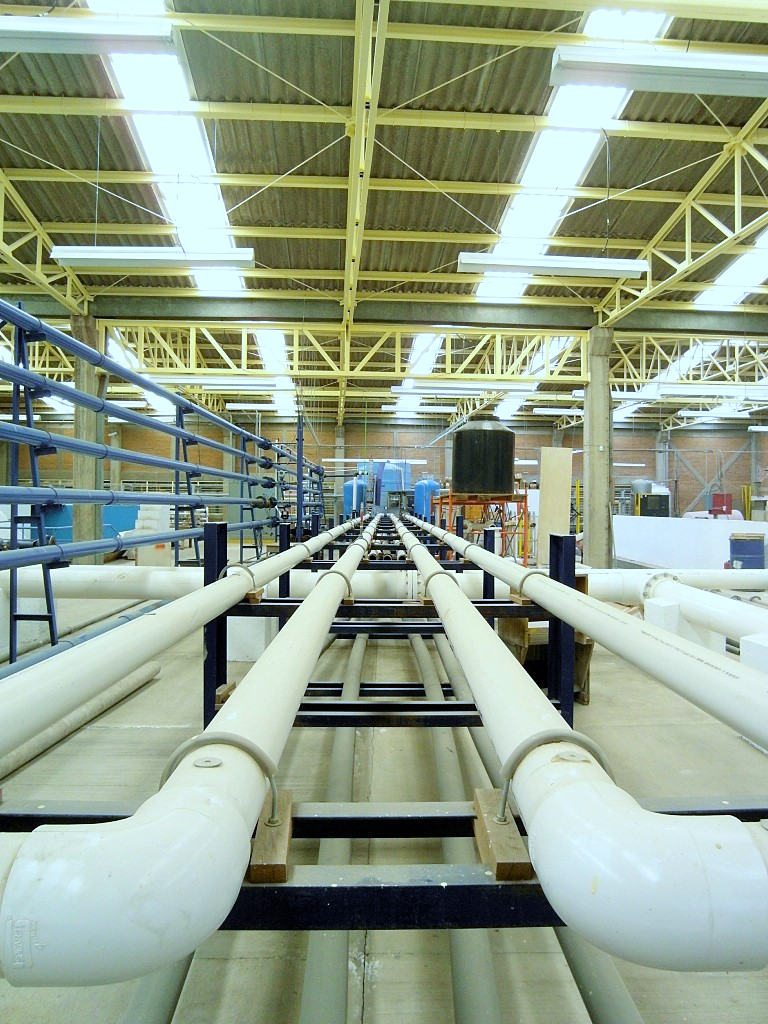
We are looking to reduce the uncertainties by applying deep learning (DL) and machine learning (ML) methods taken from AI. These ML and DL methods are going to mimic the acumen and experience of the experts who need to make an inference based on field characteristics. Their expertise in inputting parameters will be used in setting the simulator. If the results of the simulation are not close to the field measurements, then they will be deemed unsatisfactory and the parameters will need to be tweaked and run again. The goal of these AI methods is to obtain a set of input parameters that will produce simulation results that mirror the quantities measured on field as accurately as possible. By introducing this method, production costs are expected to be minimized due to the reduction of simulation time.
Written by:
Jaime Klapp (Instituto Nacional de Investigaciones Nucleares)